Difference Between Machine Learning and Artificial Intelligence
Data:
26 Marzo 2024
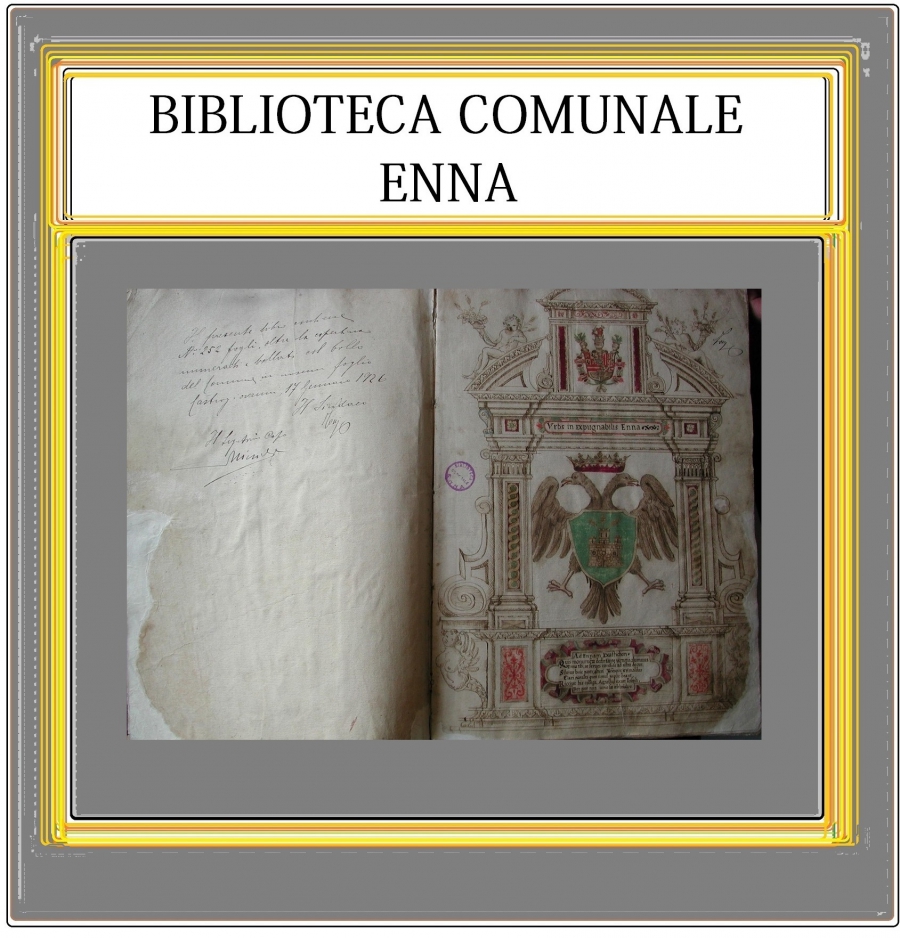
Machine Learning vs AI: Similarities and Differences
Software engineering is about more than just writing code; it’s the art and science of designing, developing, and maintaining software systems that power our societies. It’s the discipline that provides the foundational technology for the modern digital world, from mobile applications to the complex algorithms that drive AI. Whether you use AI applications based on ML or foundation models, AI can give your business a competitive advantage. AI, however, can be used to solve more complex problems such as natural language processing and computer vision tasks. To explain this more clearly, we will differentiate between AI and machine learning.
On the other side, natural language processing is improving customer service and IT management. And deep neural networks are using image recognition to improve search for a variety of industries. Set and adjust hyperparameters, train and validate the model, and then optimize it.
Key differences between Artificial Intelligence (AI) and Machine learning (ML):
Machine learning algorithms, then, can be regarded as the essential building blocks of modern AI. Machine learning finds a pattern or anomaly amongst the noise of data and finds paths to solutions within a time frame that humans would not be capable of. They also help impart autonomy to the data model and emulate human cognition and understanding.
This AI Paper Introduces POYO-1: An Artificial Intelligence Framework Deciphering Neural Activity across Large-Scale Recordings with Deep Learning – MarkTechPost
This AI Paper Introduces POYO-1: An Artificial Intelligence Framework Deciphering Neural Activity across Large-Scale Recordings with Deep Learning.
Posted: Mon, 30 Oct 2023 10:26:23 GMT [source]
Deep learning models require little to no manual effort to perform and optimize the feature extraction process. In other words, feature extraction is built into the process that takes place within an artificial neural network without human input. Since deep learning algorithms also require data in order to learn and solve problems, we can also call it a subfield of machine learning. The terms machine learning and deep learning are often treated as synonymous. Explaining how a specific ML model works can be challenging when the model is complex. In some vertical industries, data scientists must use simple machine learning models because it’s important for the business to explain how every decision was made.
Why Google
It allows systems to recognize patterns and correlations in vast amounts of data and can be applied to a range of applications like image recognition, natural language processing, and others. Today, artificial intelligence is at the heart of many technologies we use, including smart devices and voice assistants such as Siri on Apple devices. As a contrast to supervised learning, unsupervised learning does not require its input data sets to be labeled. And since it’s datasets will not be labeled, it will not be as accurate as supervised learning.
Generative AI can perform tasks like analyze the entire works of Charles Dickens, JK Rollins or Ernest Hemingway and produce an original novel that seeks to simulate these authors’ style and writing patterns. The world of Machine Learning has a vast kingdom of its own unique capabilities, domains, and powers. Under Machine Learning, algorithms are trained on how to systematize and make predictions on the basis of patterns and statistical implications with the help of pre-existing data. AI/ML solutions are tailor-made for tackling intricate tasks that require precise outcomes based on a wealth of acquired knowledge.
A supervised learning machine algorithm is trained on a small data set that needs to be labeled by a human. It will learn from it and try to apply solutions on more massive datasets. The training dataset will provide the fundamental algorithm problem, data points, and the solution. Machine learning is an application of AI that is based around the idea that we can give machines data, and allow them to learn for themselves.
By utilizing multiple forms of machine learning systems, models, algorithms and neural networks, generative AI offers a new foray into the world of creativity. Machine learning (ML) is a technique used to help computers learn tasks and actions using training that is modeled on results gleaned from large data sets. Despite scaremongering projections that millions will need to switch occupations as robots and algorithms take over specific tasks once done by humans, most analyses project job gains as a result of AI, machine learning, and deep learning. Gartner predicts that in the US AI will create two million net-new jobs by 2025, as companies expand to absorb the new productivity. Armed with vast amounts of data, these machines navigate through complex patterns, masterfully weaving together astonishingly realistic creations that rival, and at times surpass, human ingenuity. Simultaneously, machine learning unveils its enchanting prowess, crafting algorithms that empower machines to gather knowledge from massive datasets effortlessly.
This is the realm of accurate predictions and informed decisions, as patterns and trends reveal themselves. It helps to foresee sales, customer behaviour, market trends, and more, empowering businesses to optimise operations, skyrocket customer satisfaction, and outshine competitors. Generative AI and machine learning join forces to create an unstoppable duo that is reshaping the computer science landscape in extraordinary and awe-inspiring ways.
Read more about https://www.metadialog.com/ here.
Ultimo aggiornamento
3 Novembre 2024, 18:23